System Introduction
The abnormal detection system utilizes the Empirical Modeling technique, a machine learning method, to learn the relationship patterns among various sensors of the monitored equipment using historical operational data. It creates optimized predictive models for each equipment to detect anomalies and faults. The models compare predicted values for each sensor of the equipment with real-time operational values, providing early alerts when deviations exceed the allowable range, indicating abnormal conditions of the equipment.
Main functions
- Train empirical model with equipment’s historical data (Machine Learning)
- Estimated, deviation and dynamic predicted range
- Compare estimated and actual value to create early warning
- View and analyze early warning information
- Diagnostic Rule function
- Interface with real-time information system platform
Use Case
Steam Turbine lube oil supply unit problem detected case
- An issue has occurred with the customer's steam turbine lubricating oil supply unit, causing a drop in header pressure to 0.2 kg/cm2
- At the same time, the steam turbine bearing temperature has increased more than 3 degrees. triggering an early warning
- Early warning triggered by system to inform operator and user has recognized the trouble.
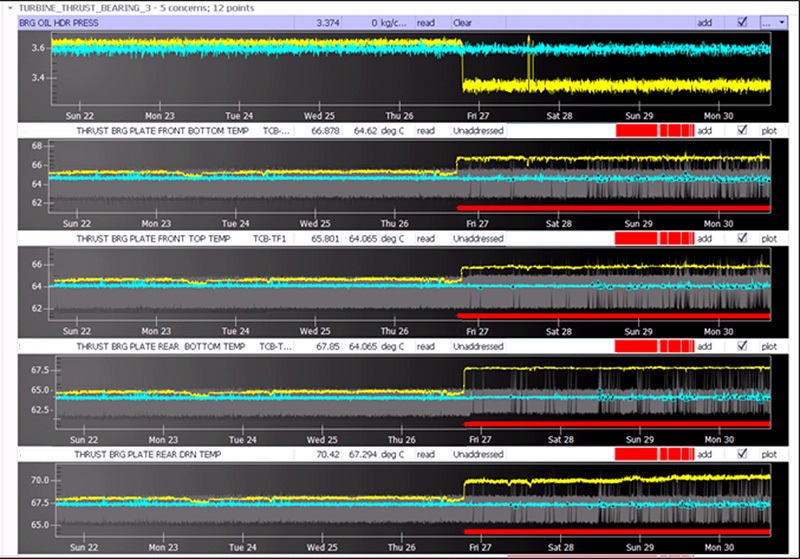
[Accident Detection Prediction Chart Example]